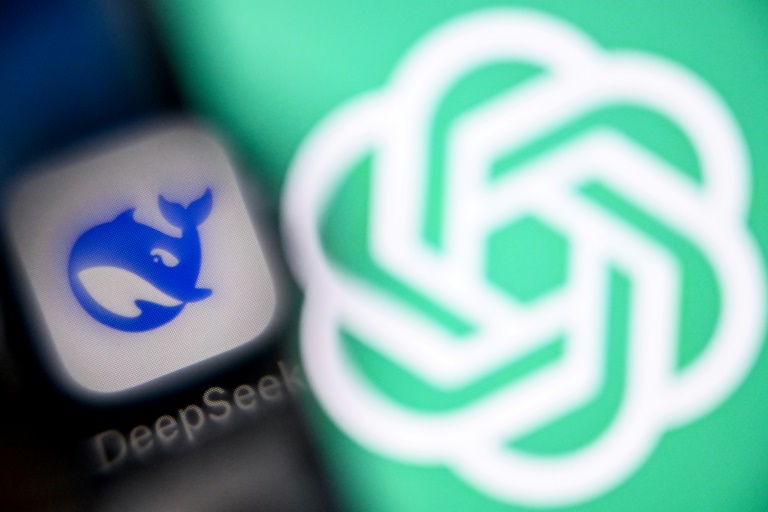
As generative artificial intelligence systems grow more powerful and influential, even their creators acknowledge a critical gap in understanding: they don’t fully grasp how these digital minds arrive at their decisions.
While AI technologies such as ChatGPT, image generation tools, and autonomous agents are being hailed as transformative forces in society and industry, researchers are raising concerns about the opacity of these systems’ inner workings. This challenge arises mainly due to the complex structure of modern AI models, particularly large language models and deep neural networks, which operate with millions or even billions of interconnected parameters.
A few years ago, studying the internal logic of AI systems was a niche academic endeavor with limited research focus. Today, it has rapidly evolved into one of the most urgent and active areas of investigation. Researchers seek to peel back the layers of these digital minds, attempting to trace the logic of their decisions and diagnose potential biases, errors, or harmful behaviors.
One major difficulty lies in the nature of how such AI systems learn. Unlike traditional software, which follows a clear set of coded instructions, generative AI models ‘learn’ patterns through massive datasets and adjust themselves based on probability distributions. This results in a kind of statistical intuition rather than transparent reasoning, making it profoundly difficult—even for experts—to predict or interpret specific responses.
This lack of transparency has sparked debates about the ethics and safety of deploying such systems at scale. If scientists and engineers do not fully comprehend how their creations function internally, can they truly be trusted in high-stakes environments such as medicine, law, or autonomous vehicles?
Nonetheless, the AI research community is urgently developing tools to probe these digital thought patterns. Techniques such as feature visualization, interpretability maps, and circuit tracing within neural networks are being refined to open the ‘black box’ of artificial cognition. These efforts aim not only to increase trust and safety in AI systems but also to inform the next generation of smarter and more accountable machine intelligence.
In summary, while the capabilities of AI are advancing at remarkable speeds, understanding exactly how these artificial minds operate remains one of the greatest scientific and ethical challenges in the field today.
Source: https:// – Courtesy of the original publisher.