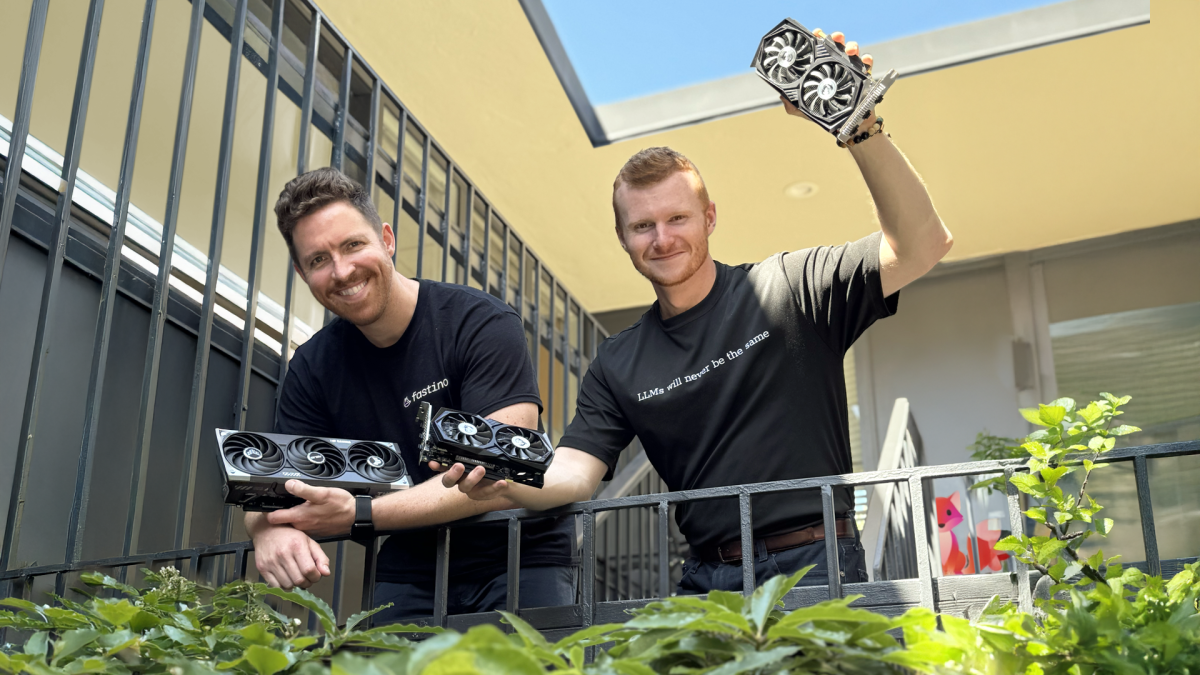
In an industry dominated by ever-escalating model sizes and hardware costs, Fastino, a rising technology startup, is challenging the norms of artificial intelligence development. While tech giants such as OpenAI, Google, and Meta tout the scale of their trillion-parameter AI systems—demanding vast GPU clusters and substantial energy consumption—Fastino has adopted a more resource-efficient methodology focused on compact, high-performance models.
Fastino’s philosophy centers around building smart, lightweight AI systems that deliver competitive performance without the need for massive compute infrastructure. This strategy not only helps to democratize access to advanced AI technology by reducing its operational costs, but also addresses growing environmental concerns associated with high-energy AI training processes.
The company achieves this through a combination of algorithmic optimization, model architecture refinement, and leveraging new hardware efficiencies. According to sources close to the company, Fastino is demonstrating that more efficient models can serve a wide range of commercial and enterprise use cases—such as language understanding, image recognition, and predictive analytics—without requiring the extensive technical debt associated with scaling traditional deep learning models.
While details about Fastino’s proprietary technology remain under wraps, its alternative method is attracting attention from both investors and researchers who are increasingly critical of the sustainability of current AI trajectories. As AI adoption grows, so too does the need for accessible and efficient solutions capable of running on everyday devices rather than exclusive data centers.
Fastino’s approach could mark a significant shift in the industry, emphasizing optimization over brute-force computation. By demonstrating that smaller, more strategic design can rival or even surpass models built purely for scale, Fastino may be paving the way for the next generation of smart, sustainable AI.
Source: https:// – Courtesy of the original publisher.