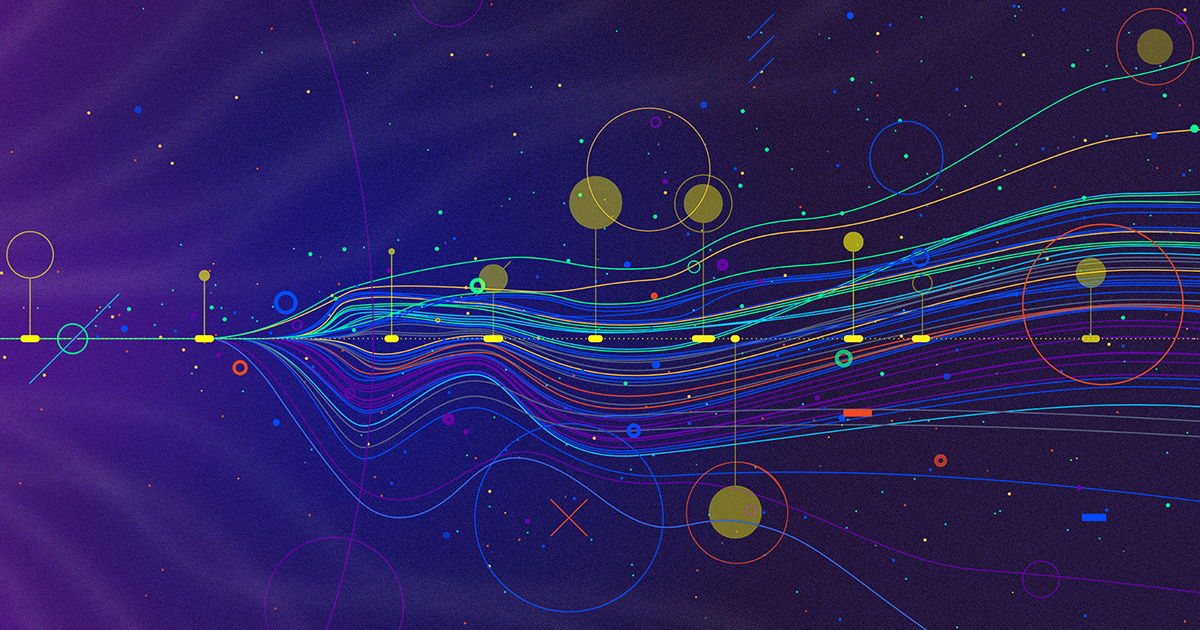
Artificial intelligence is increasingly playing a foundational role in reshaping the way science and mathematics are conducted. From generating new hypotheses to uncovering patterns in massive datasets, AI systems are not only accelerating the pace of discovery but also redefining how knowledge itself is produced.
In scientific research, AI tools can now design and run experiments, make predictions based on data, and even suggest novel theories that may not occur to human researchers. In mathematics, machine learning models are helping to unearth complex relationships and conjectures that were previously hidden in high-dimensional data spaces.
These advancements are sparking deep philosophical discussions within the scientific community about the nature of scientific methodology. Traditionally, scientific discovery hinged on human intuition, creativity, and reasoning. But as intelligent algorithms display an increasing capacity for problem-solving and pattern recognition, researchers are reconsidering what constitutes understanding and explanation in science.
At the same time, the role of the scientist is evolving. Instead of solely relying on direct analysis and experimentation, scientists are becoming system designers and interpreters—building and guiding AI systems while interpreting their outputs in meaningful ways.
As this transformation continues, it raises crucial questions about transparency, accountability, and the ethical use of AI in research. Ensuring that AI-driven insights remain interpretable and reproducible will be critical for maintaining trust in scientific results.
Ultimately, the integration of artificial intelligence into science and mathematics is not just a technological shift—it is a profound reconceptualization of how we explore, understand, and explain the natural world.
Source: https:// – Courtesy of the original publisher.